Introduction:
AI is changing how companies hire people. About 8 out of 10 businesses use AI to find and choose new talent. With new kinds of AI coming out, even more companies will probably start using it.
But there are worries about AI. New laws like the EU AI Act and New York's AI Bias are trying to stop AI from being unfair. Companies need to be careful when using AI to make sure it's fair and works well.
This blog will talk about a way to check if AI is safe to use, and how GCC can implement it in finding the right talent.
Defining and Distinguishing Between Recruitment Automation and AI:
The blurred lines between recruitment automation and artificial intelligence often lead to misconceptions. While both technologies aim to enhance the hiring process, they serve distinct purposes and carry different implications.
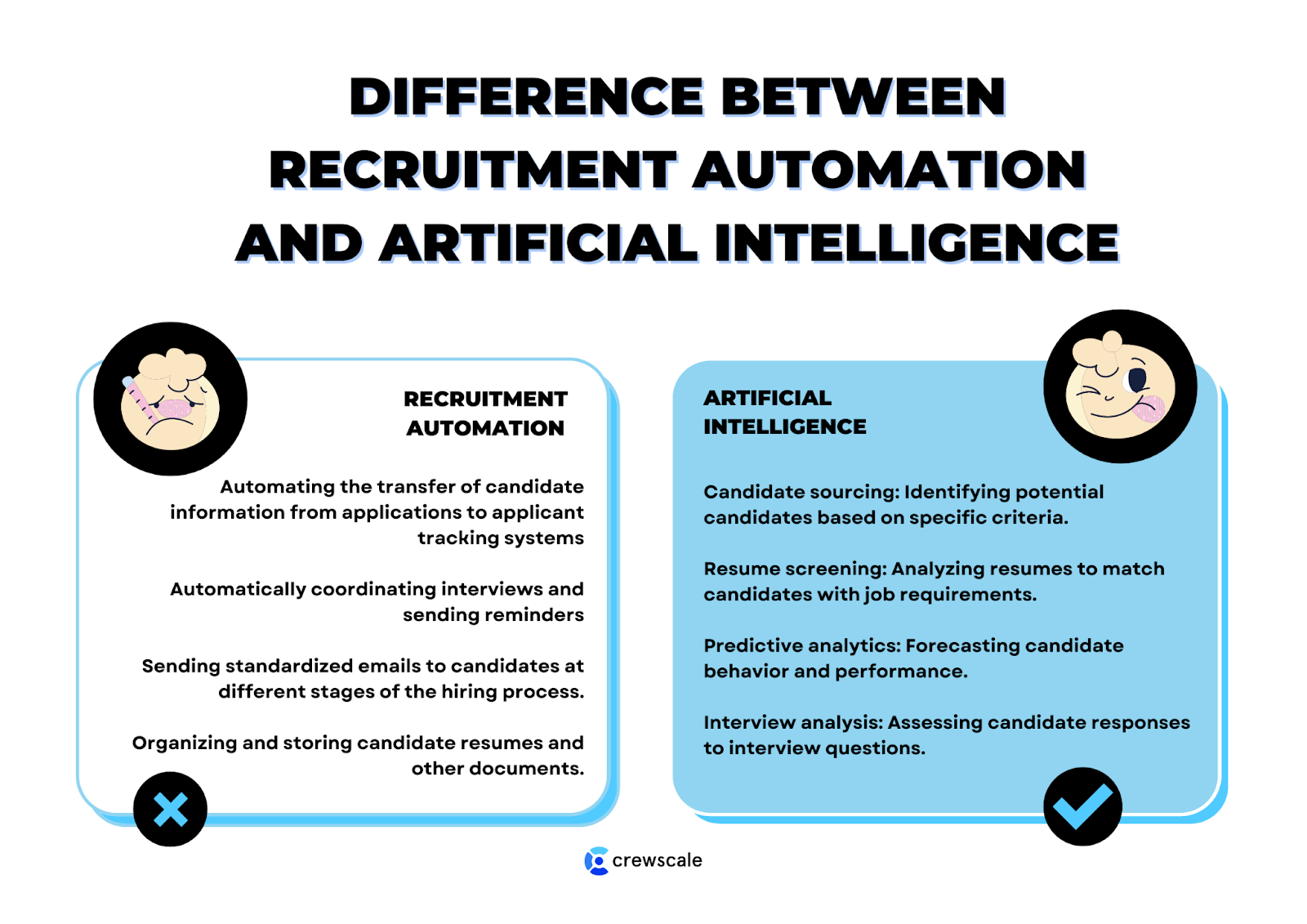
Recruitment Automation:
Recruitment automation focuses on task optimization. It involves using technology to execute repetitive, time-consuming tasks, freeing up recruiters to concentrate on strategic initiatives. These tasks typically include:
- Data entry: Automating the transfer of candidate information from applications to applicant tracking systems.
- Scheduling: Automatically coordinating interviews and sending reminders.
- Emailing: Sending standardized emails to candidates at different stages of the hiring process.
- Document management: Organizing and storing candidate resumes and other documents.
By automating these routine functions, recruitment automation significantly improves efficiency and reduces the risk of human error.
Artificial Intelligence:
Artificial intelligence goes beyond automation by introducing learning and decision-making capabilities. It leverages algorithms and machine learning to analyze vast amounts of data, identify patterns, and make predictions. In recruitment, AI can be applied to:
- Candidate sourcing: Identifying potential candidates based on specific criteria.
- Resume screening: Analyzing resumes to match candidates with job requirements.
- Predictive analytics: Forecasting candidate behavior and performance.
- Interview analysis: Assessing candidate responses to interview questions.
AI's ability to learn and adapt over time enables it to provide increasingly accurate insights and recommendations.
To make sure AI is used correctly and safely in companies, we need to understand the risks and challenges associated with it. Only then can we use AI to its full potential, helping people and software work together to reach common goals.
Roadmap To Understand The Possible Problems With AI And How GCC Can Safely Use AI For Talent Acquisition:
As organizations increasingly integrate Artificial Intelligence (AI) into their recruitment processes, understanding and mitigating the associated risks becomes critical. The AI Risk Framework provides a comprehensive approach to ensuring that AI systems are not only effective but also safe, fair, and aligned with organizational values.
This framework, adapted from the National Institute of Standards and Technology (NIST) and the World Economic Forum, outlines seven key domains to consider when deploying AI in recruitment.
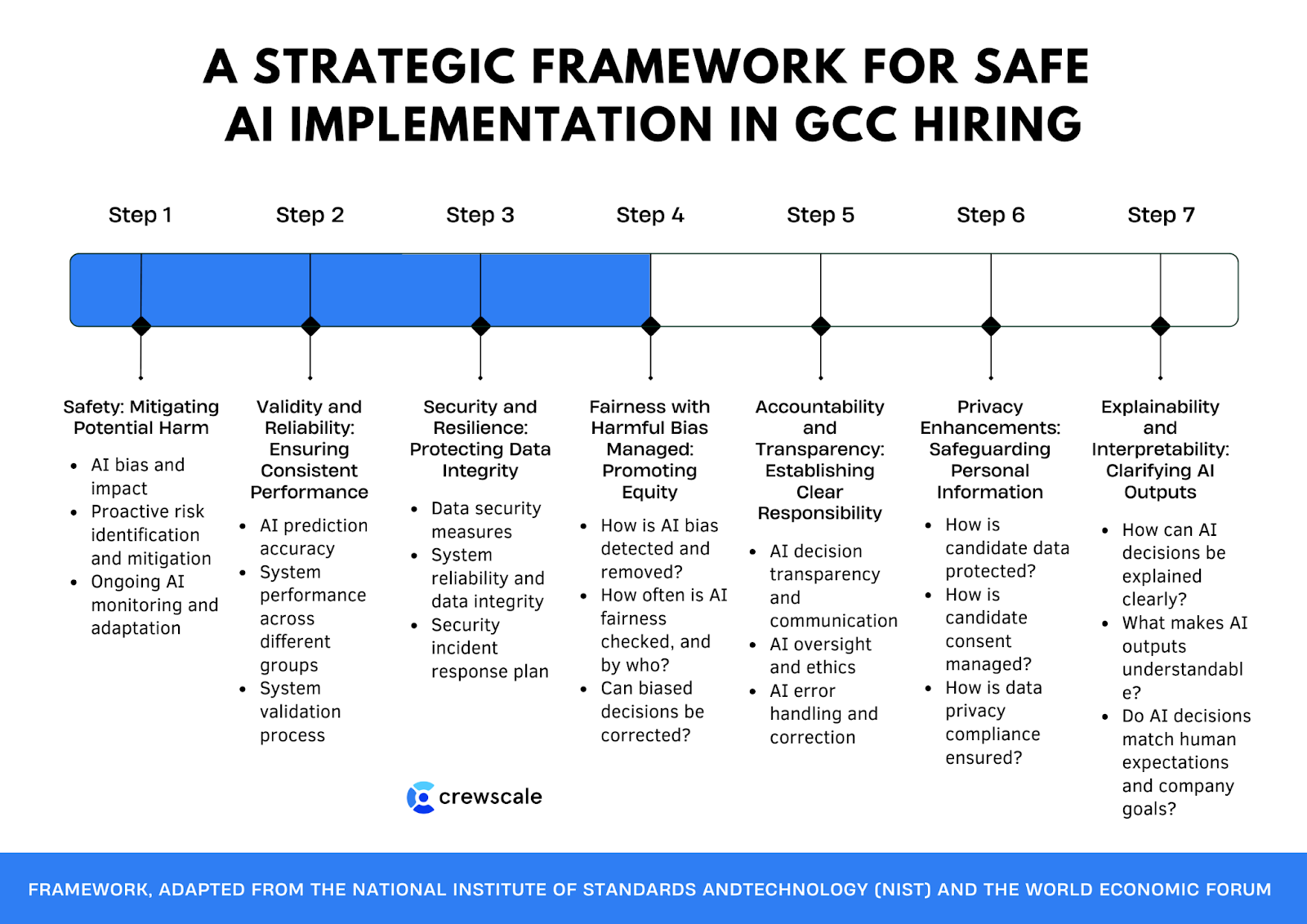
1. Safety: Mitigating Potential Harm
The foremost concern when implementing AI in recruitment is safety. AI systems, if not carefully managed, can pose significant risks, such as unintentional discrimination or privacy breaches. It is crucial to evaluate the potential for harm by analyzing how AI decisions could negatively impact individuals or groups. This involves understanding the full scope of AI's impact on recruitment, including unintended consequences, and establishing safeguards to prevent harm.
Key Considerations:
- How might AI decisions disproportionately affect certain demographics?
- What measures are in place to identify and address potential harms before they manifest?
- Are there mechanisms to monitor AI's impact over time and adjust its functioning as needed?
2. Validity and Reliability: Ensuring Consistent Performance
For AI to be trusted in recruitment, it must consistently perform its intended tasks with accuracy. Validity refers to the system's ability to achieve the desired outcomes, while reliability pertains to its performance under varying conditions. Inconsistent results or deviations from expected behavior can undermine confidence in the technology and lead to suboptimal hiring decisions.
Key Considerations:
- Does the AI system accurately predict candidate success based on relevant data?
- How does the system perform across different candidate pools and under different conditions?
- What testing protocols are in place to regularly validate the system's accuracy and consistency?
3. Security and Resilience: Protecting Data Integrity
AI systems in recruitment deal with vast amounts of sensitive data, including personal and professional information about candidates. Ensuring that this data is secure is paramount. Additionally, the system must be resilient, and able to function effectively even in the face of adversity, such as cyber-attacks or system failures.
Key Considerations:
- What encryption methods and security protocols are employed to protect candidate data?
- How does the system ensure continuity and data integrity during technical disruptions?
- Are there robust incident response plans in place to address security breaches?
4. Fairness with Harmful Bias Managed: Promoting Equity
AI systems can inadvertently perpetuate or even amplify biases present in historical data. Ensuring fairness requires implementing controls to identify, mitigate, and monitor harmful biases within the AI’s algorithms. This is especially important in recruitment, where biases can significantly impact diversity and inclusion within an organization.
Key Considerations:
- What methods are used to detect and eliminate bias in AI models?
- How frequently is the AI system audited for fairness, and by whom?
- Are there processes for rectifying decisions that were influenced by bias?
5. Accountability and Transparency: Establishing Clear Responsibility
Transparency in AI operations is essential for accountability. Stakeholders must understand how AI decisions are made, who is responsible for these decisions, and what measures are in place to ensure accountability. This transparency not only builds trust but also ensures that any issues can be quickly identified and addressed.
Key Considerations:
- How are AI decision-making processes documented and communicated to stakeholders?
- Who is responsible for overseeing AI operations and ensuring compliance with ethical standards?
- What protocols exist for investigating and rectifying erroneous or biased AI decisions?
6. Privacy Enhancements: Safeguarding Personal Information
AI systems in recruitment often require access to personal data, making privacy protection a critical concern. AI systems must respect privacy and uphold the dignity of candidates by ensuring that personal information is used appropriately and protected from misuse.
Key Considerations:
- How is personal data anonymized or pseudonymized to protect candidate privacy?
- What consent mechanisms are in place to ensure candidates are informed about how their data will be used?
- How does the system comply with relevant data protection regulations (e.g., GDPR, CCPA)?
7. Explainability and Interpretability: Clarifying AI Outputs
AI systems must produce outputs that are not only accurate but also understandable. Explainability and interpretability are critical in ensuring that stakeholders can trust AI decisions and use them effectively in recruitment. This involves making the AI's decision-making process transparent and ensuring that the reasons behind AI recommendations are clear and justifiable.
Key Considerations:
- How are AI decisions explained to recruiters and other stakeholders?
- What tools or methods are used to make AI outputs interpretable?
- Are AI decisions aligned with human expectations and organizational goals?
Implementing the AI Risk Framework: A Strategic Approach:

Step 1: Ask Key Questions Across Seven Domains
Start by posing critical questions within each of the seven domains outlined above. These questions should be tailored to your organization’s specific needs and the context in which AI will be used. This step is essential for identifying potential risks and areas that require further examination.
Step 2: Evaluate the Risk Level
Once the questions have been addressed, evaluate the level of risk associated with AI implementation in each domain. Categorize these risks into three levels:
- Unacceptable Risk: Requires immediate mitigation or may necessitate abandoning the AI system in its current form.
- Mitigation Required: Risks that can be managed with appropriate controls and adjustments.
- Acceptable Risk: Risks that are minimal and within the organization’s risk tolerance.
Step 3: Determine Next Steps
Based on the risk evaluation, develop a strategic plan to address the identified risks. This may involve refining the AI system, implementing additional controls, or training staff on how to interpret and manage AI outputs. The goal is to ensure that AI enhances the recruitment process without compromising safety, fairness, or transparency.
Roadmap for Transforming Recruitment: From Conventional to AI-Driven Processes:
The roadmap depicted in the image outlines a clear transition from traditional recruitment methods to an AI-enhanced recruiting platform. This roadmap is designed to streamline the hiring process, improve efficiency, and ensure more accurate candidate selection. Below is a detailed breakdown of the process flow from both the conventional recruiting model and the AI-driven recruiting platform.

Step 1: Job Advertising
- Objective: Attract potential candidates to apply for open positions.
- Action: Advertise job openings through various channels (job boards, company website, social media).
This step remains consistent across both conventional and AI-driven models. However, the subsequent stages differ significantly in how candidates are screened and selected.
Step 2: Screening Selection
Conventional Model:
- Action: Candidates apply by submitting their documents, such as CVs and cover letters. The initial screening may include personality tests or knockout questions to filter out unsuitable candidates.
- Manual Screening: Recruiters manually review the submitted documents, scoring assessments based on predetermined criteria. This process is labor-intensive and time-consuming.
AI-Driven Model:
- Action: Candidates submit their documents, similar to the conventional model. However, the AI platform enhances this step by incorporating the job description as a key input for analysis.
- AI Parsing and Analyzing: The AI system automatically parses and analyzes the submitted documents, cross-referencing them with the job description and other assessments. This allows for more accurate and unbiased screening of candidates.
- Automated Chatbot Interview: AI platforms may also include an automated chatbot interview, where candidates are asked pre-screening questions, personality tests, or mini-cases. The responses are fed back into the AI system for further analysis and learning.
Step 3: Interview Process
Conventional Model:
- Manual Interview Selection: Based on the manual screening, recruiters shortlist candidates for phone interviews or email questionnaires.
- Action: Recruiters conduct phone interviews or send questions via email to assess the candidates further.
- Output: The results of these interviews lead to a ranked list of candidates, who are then shortlisted for face-to-face interviews.
AI-Driven Model:
- Automated Recommendation and Ranking: After analyzing all inputs, including the automated interviews, the AI system ranks the candidates and provides recommendations for the next steps.
- Human Decision-Making: The final decision to proceed with interviews is made by human recruiters, who review the AI-generated rankings and recommendations.
- Output: Candidates who pass the AI screening are automatically ranked based on their suitability for the role, allowing recruiters to focus on the highest-potential candidates.
Step 4: Face-to-Face Interview
- Conventional Model: Selected candidates from the manual process proceed to face-to-face interviews.
- AI-Driven Model: Candidates who have been ranked and recommended by the AI system are interviewed by recruiters, focusing on final selection.
Key Differentiators Between Conventional and AI-Driven Recruitment:
- Efficiency Gains: The AI-driven model significantly reduces the time spent on manual document screening and initial interviews by automating these tasks.
- Improved Accuracy: AI parsing and analysis lead to more consistent and accurate candidate evaluations, reducing the likelihood of human error or bias.
- Data-Driven Insights: The AI model provides data-driven insights and recommendations, which can enhance the overall quality of hires.
- Scalability: The AI system can process a large number of applications simultaneously, making it ideal for organizations with high-volume recruitment needs.
This roadmap outlines the journey from a conventional labor intensive recruiting process to an AI-driven model that enhances efficiency, accuracy, and decision making.
Conclusion:
By adopting AI in recruitment, organizations can streamline their processes, reduce biases, and focus on strategic decision-making, ultimately leading to better hiring outcomes and a more robust talent pool.